In its commitment to nurturing the already burgeoning growth of student-led data-intensive research at Johns Hopkins, The Institute for Data Intensive Engineering and Science (IDIES) has the privilege of providing financial support for exemplary students to complete 10-weeks of summer research through our Summer Student Fellowship (SSF) initiative.
Undergraduate students have the chance to receive up to $6,000 in grant funding for their own eligible data-intensive research projects.
Eligible research projects for the Summer Student Fellowship must:
(a) involve data science and data-intensive techniques
(b) have support and mentorship (via a letter of recommendation) from a current IDIES faculty member
(c) be entirely student-led and designed
Without further ado, allow us to introduce this year’s SSF cohort and their projects for summer 2023:
More detailed information about each fellow and their project can be found below or by clicking on an above headshot or project title.
Please join us in congratulating our 2023 Summer Student Fellows.
2023 SSF Winners
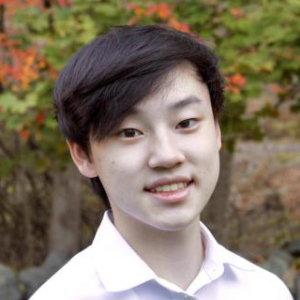
Jay Kim
First-year Student in Materials Science & Engineering, Computer Science
Jay Kim is a freshman attending Johns Hopkins University, double majoring in Materials Science & Engineering (MSE) and Computer Science. Originally from New York, Kim has high school experience in TEM research and is currently continuing work in the same branch with the Taheri Group in the department of MSE.
PROJECT:
Intelligent Microscopy Methods for Characterizing Intermediate States Using Electron Energy Loss Spectroscopy (EELS)
Advised by: Professor Mitra Taheri
“Advances in Transmission Electron Microscope (TEM) resolution, precision, and collection rates have greatly improved our ability to probe complex structures and changes in materials. Machine learning frameworks, such as variational auto-encoders are powerful tools that enhance the processing and characterization of the complex signals received from the instruments. Our auto-encoder will be trained using a set of handwritten digits provided by the MNIST database. We aim to train the auto-encoder to cluster and classify the different digits and image the latent space. We will then transition into a SrFeO3 TEM Electron Energy Loss Spectroscopy (EELS) dataset. We hope to identify, cluster, and classify transient intermediate states of observed chemical reactions and produce higher quality de-noised images.”
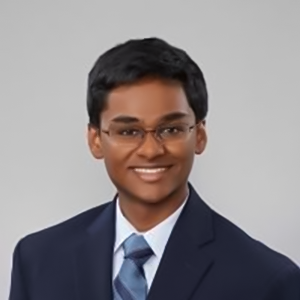
Sampath Rapuri
First-year Student in Biomedical Engineering,
Computer Science
Sampath Rapuri is a first-year student pursuing a dual degree in Biomedical Engineering and Computer Science. He is interested in developing computational pipelines that can improve the precision, efficacy, and outcomes of care delivered to critically ill patients. After graduation, he plans to pursue an MD-PhD and establish his own research lab. In his free time, Sampath enjoys playing badminton and gardening.
PROJECT:
Development and External Validation of a Machine Learning Model for Pulmonary Embolism
Advised by: Professor Robert D. Stevens
“Pulmonary embolism (PE) is a severe and frequent complication in critically ill patients. While several clinical risk scores have been proposed, there is still an unmet need for accurate methods to predict the likelihood of developing PE. Accurate prediction is crucial for timely diagnosis and treatment, as untreated acute PE has a high mortality rate. On the other hand, diagnosed and treated PE has a significantly lower mortality rate. Therefore, a data-driven computational model that utilizes available physiological parameters has the potential to improve accuracy and help clinicians identify and treat PE in ICU patients more effectively. The objective of this study is to develop and externally validate a data-driven computational model for PE prediction in critically ill patients and compare its performance with currently available ICU PE scoring systems. “
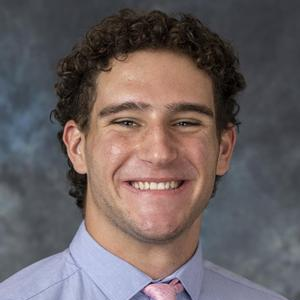
WIlliam Shiber
Second-year Student in Applied Mathematics & Statistics
William Shiber is a second-year student pursuing a BS/MS in Applied Mathematics and Statistics with a focus on Statistics and Statistical learning. While originally from northern New Jersey, Shiber has spent much of his time in in New Hampshire.
PROJECT:
AFLUX@JHU: Materials Search-API for the JHU aflow.org Data Repositories
Advised by: Professor Corey Oses
“This project aims to design and implement AFLUX@JHU: a materials search-API for the aflow.org data repositories that are generated and housed at Johns Hopkins. The aflow.org framework is high-throughput materials simulation software used to discover novel materials and predict material properties. Our goal is to implement an API to allow machine learning access to this data, which consists of over 3.5 million stored entries. AFLUX@JHU will include tensor-like filterability and atom- and species-specific properties filterability to optimize workflows. AFLUX@JHU will provide access to a growing compendium of highly-filterable materials data, not only easing the user experience by placing the computational burden server-side but also making such searches possible for queries wrangling millions of entries.”
IDIES is a major interdisciplinary program, a large, diverse effort, where faculty and students work together to solve data-intensive problems, from genes to galaxies to materials science and urban planning.
The IDIES funding programs seek to encourage new ideas and grow these efforts across the university, while giving JHU researchers and students the opportunity to expand their data science analysis and projects to the next level.