Seed Funding Awardees
Recipients of the IDIES Seed Funding Awards
The IDIES Seed Funding Program Awards are competitive awards of $25,000. The Seed Funding initiative provided funding to the following data-intensive computing projects because they (a) involve areas relevant to IDIES and JHU institutional research priorities, (b) are multidisciplinary; and (c) build ideas and teams with good prospects for successful proposals to attract external research support by leveraging IDIES intellectual and physical infrastructure.
Abstracts from previous Seed Funding recipients’ projects are available below.
Spring 2023
PI: G. Caleb Alexander, MD (Bloomberg School of Public Health)
Co-I: Anqi Liu
“We propose to develop state-of-the-art computer-vision software to rapidly and efficiently identify compelling visual artifacts in the Opioid Industry Documents Archive (OIDA), a highly innovative document collection focused on the U.S. opioid epidemic.
Specifically, we have three objectives:
To improve OIDA’s current Python code for extracting images from PowerPoint and Excel documents to filter out the smallest, least meaningful images
To develop new code that uses computer vision to detect images within raster PDFs, which comprise the vast majority of documents in the OIDA dataset Z
To develop a supervised machine-learning pipeline to select from among the extracted images those that are most meaningful for sharing in an online gallery”
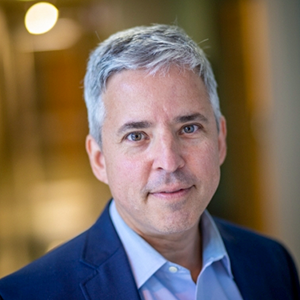
Co-Is: Joseph P. Angelo, Anissa Elayadi, and Robert L. Wilson
“Photoplethysmography (PPG) is an optical technique that senses blood volume changes from the arterial pulse signal and is used worldwide to monitor heart rate and blood oxygenation (e.g. commercial pulse-oximetry). While robust, it requires constant sensor contact to the skin. Remote PPG (rPPG) via RGB cameras utilizes the same basic principles but enables patient monitoring from a distance. We developed rPPG extraction methods through analysis of our large size sensor data (DSLR). Funding from IDIES will allow us to translate these methods to complete analysis of our small size sensor data (smartphone), optimizing extraction of non-contact blood oxygen saturation and blood pressure from RGB data. Our approach using off-the-shelf RGB sensors promises a scalable best-case tool for remote vital signs.”
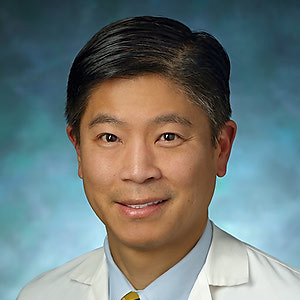
Co-Is: Co-Pierre Georg
“Modern software development involves collaborative efforts and re-use of existing software packages and libraries, to reduce the cost of developing new software. However, package dependencies expose developers to the risk of contagion from bugs or other vulnerabilities that may cost billions of dollars. This project will model the maintainers’ decisions to create dependencies among software libraries in an equilibrium strategic network formation game. After estimating the parameters of such model using data from https://libraries.io, we can quantify and understand the externality imposed by such dependencies in terms of contagion risk from bugs or other vulnerabilities. This analysis will provide a measure of systemic risk for a software ecosystem.”
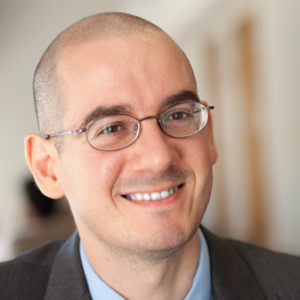
Co-I: Sara Thoi
Lithium-sulfur batteries offer a promising alternative to conventional Li-ion technology, swapping the intercalation process for multi-electronic redox chemistry. Unfortunately, these reactions are not fully reversible in common electrolytes resulting in degradation of the cathode and insulation of the anode with sulfur-containing precipitate, limiting capacity and thus overall cyclability. Oses and Thoi look at address this “shuttle” effect by designing new high-entropy anchors that immobilize the lithium polysulfide species to the cathode. The team will employ data-driven thermodynamic modeling to screen the vast search space of candidates afforded by a high-entropy design.
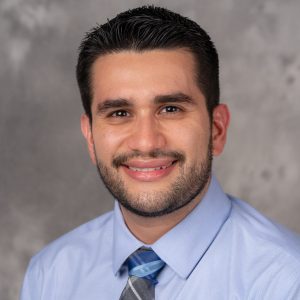
Spring 2022
Neurological outcomes of ischemic stroke (IS) have substantially improved due to advances in the available treatment options. However, these treatments are highly time-sensitive and are often delayed because symptoms may be quite variable and of uncertain significance, especially for untrained observers. We hypothesize that quantifiable abnormalities in facial expression, eye movements, and speech (phenotypic features) are detectable in all stroke patients, and that these features can be extracted using computational algorithms applied to smartphone video recordings of facial expression and speech. Our aim is to create a system for IS detection and severity assessment based on computational analysis of these phenotypic features. We also aim to develop a prognostic system to determine the clinical outcome of IS from phenotypic signals.

Co-Is: Nam Q. Le and Jarod Gagnon
In collaboration with researchers at APL, Drs. Nam Q. Le and Jarod Gagnon, the Clancy group will use an “on-the-fly” active learning approach within the umbrella of machine learning to study a novel additive manufacturing process to create gallium nitride thin films. This approach combines the accuracy of a first-principles, ab initio, method with the orders of magnitude faster execution speed of using an empirical force field MD, essentially the best of both worlds. It will also us to capture the details of the formation of gallium nitride by a chemical reaction in the liquid phase and model the subsequent crystallization process with atomistic precision. Being able to essentially ‘print’ this material should have implications for energy transmission and efficiency.

Co-Is: Marilyn Albert, Milap Nowrangi, and Hannah P. Cowley
More than 5 million people suffer from Alzheimer’s Disease (AD) in the US alone with additional, untold impacts on caregivers. An astonishing, yet underappreciated, aspect of AD are the moment-to-moment fluctuations in cognitive ability, including ‘positive’ periods of uncharacteristically coherent communication and cognitive abilities. These ‘episodes of lucidity’ are rare, unpredictable and yet undeniably precious. Interestingly, context may play a critical role in triggering improvements in cognition. For example, a nostalgic smell or a wedding song can elicit periods of heightened cognition even for patients deep in cognitive decline. This suggests the brain still has cognitive capacity in reserve despite being rarely accessible. Can these hidden abilities be unlocked? Here, we aim to develop a mobile technology platform to integrate psychometric tests, wearable health sensors, and caregiver reports to collect multidimensional data regarding the features and predictors of cognitive fluctuations. Using machine learning and data mining, we aim to exploit these insights to improve cognition on-demand.
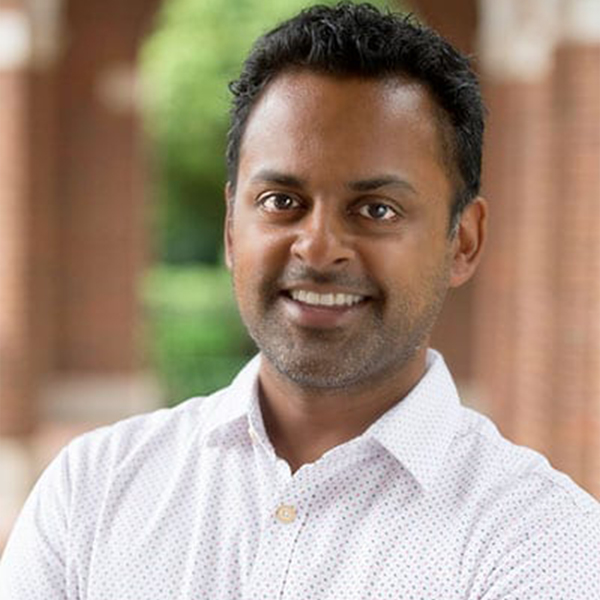
Co-Is: Bryan K. Ward, John Patrick Carey, and John Ratnanather
Hearing and balance disorders affect people of every demographic worldwide, interfering with quality of life and potentially leading to an array of negative health outcomes. Work from colleagues at Johns Hopkins and elsewhere have demonstrated strong links between hearing and balance dysfunction and dementia, depression, and reduced physical function. This project will establish proof-of-concept for building a searchable database of digitized human temporal bone (inner ear) specimens that can be accessed by any scientist with an interest in hearing and balance research. Our long-term goal is to ‘democratize’ human temporal bone research to accelerate the pace of discovery of the causes of human inner ear diseases.


Co-Is: Paul Nagy, Brian Garibaldi, Scott Pilla, Jared Zook, Harold Lehmann, Jane Valentin, Daniel Berman, and James Howard
Our project builds on the Daily24 platform. We will be creating a Covid24 dashboard that helps evaluate real-time risk for Covid. This will integrate local information with user updates to their daily interactions with others via meetings and time in office buildings. The approach should help those using the react-native Covid24 App to have increased awareness of their risks. The underlying data model and analysis builds from survival models. We use AWS for the backend and will have the App available for both iOS and Android.

Spring 2021
PI: Thomas Lippincott (Computer Science)
Co-Is: Sharon Achinstein (English), Jacob Lauinger (Near Eastern Studies)
Research in the humanities often involves richly-structured datasets that are fundamentally multimodal, combining, for example, temporal and geographic information with text and images. These properties present challenges for human intelligence’s limited attention and memory, and for computational intelligence’s limited capacity for focused reasoning. This project considers empirical questions from two domains that exemplify these challenges: changes to political and moral thought across time and geography during the Colonial era, and scribal variance in cuneiform inscriptions from the Ancient Near East. By jointly representing images, transcriptions, translations, and metadata, we will determine natural clusters that emerge from neural embeddings of existing data sets, and their alignment with themes from traditional scholarship. This project ranges over the life cycle of traditional and computational research, including data curation, annotation, machine learning, and interpretation, with particular attention towards improving the traditional scholar’s ability to annotate primary sources and interact with the machine learning output.
PI: Nadia Zakamska (Physics and Astronomy)
Co-I: Tamás Budavári (Applied Mathematics and Statistics)
One of the most enduring mysteries of modern astrophysics is that of the origin of type Ia supernovae, the cosmological standard candles used in measuring the geometry of the universe. The most likely scenario is that type Ia supernovae arise as a result of a merger of two white dwarfs — compact remnants of evolution of stars like our Sun — but no candidate progenitors have yet been discovered. In this program, we will develop the necessary machine-learning tools to discover white dwarf binaries in emerging spectroscopic, photometric and astrometric datasets. This project has potential for a breakthrough in the long-standing search for type Ia progenitors.
PI: Natalia Trayanova (Biomedical Engineering and Medicine)
Co-I: Allison Hayes (Cardiology)
It is now recognized that patients recovered from COVID-19, especially those with severe COVID requiring intensive care, frequently develop long-term debilitating symptoms and hospital readmissions. Although acute cardiac complications due to COVID-19 are now described, the long-term cardiovascular (CV) complications of COVID remain unclear. It is not known what is the frequency and nature of the CV complications, or what are the predictors for developing such adverse events in the long term posthospitalization. We are now in a unique position to address this pressing clinical need. The goal of this project is to develop a real-time machine learning (ML) solution to predict long-term (1 year) adverse CV events in patients who were discharged after hospital admission for COVID-19. The warning system will be able to identify at-risk patients in real time and alert caregivers and patients, reducing mortality, ensuring the delivery of goal-oriented therapy, and providing tangible clinical decision support.
PI: Jonathan Ling (Pathology)
Co-I: Benjamin Langmead (Computer Science)
Transactive response DNA-binding protein 43kDa (TDP-43) is an RNA-binding protein known to form pathological inclusions in a variety of age-related neurodegenerative disorders. This proposal aims to mine the vast public RNA sequencing archives to uncover new mechanisms of TDP-43 dysregulation. Using an interdisciplinary approach, these findings will be validated with in silico and in vitro model systems. Insights gained from this study may reveal novel therapeutic targets and prophylactic measures to reduce the aggregation of TDP-43 and other misfolded proteins during aging
Spring 2020
PI: Natalia Trayanova (Biomedical Engineering, WSE)Co-I: David Spragg (Cardiology, SOM), Nikhil Paliwal (Alliance for Cardiovascular Diagnostic and Treatment Innovation)
To prevent recurrent ablation procedures in atrial fibrillation (AF) patients, we propose a data-driven technology that will enable a priori prediction of the success of pulmonary vein isolation (PVI). We will use existing AF patient clinical data and artificial intelligence to train predictive models for the success of PVI using catheter ablation. The overall goal of this technology is to provide clinical guidance as to which AF patients would benefit from PVI, thus maximizing the benefit of PVI while minimizing the financial costs and procedural risks of unnecessary ablation procedures.
PI: Thomas Haine (Earth & Planetary Sciences, KSAS)
Co-I: Charles Meneveau (Mechanical Engineering, WSE)
Postdoc: Miguel Jimenez-Urias (Earth & Planetary Sciences, KSAS)
The overall project goal is to apply a novel numerical procedure to Direct Numerical Simulations of canonical Rotating Stratified Flows relevant to dynamical oceanography in order to reveal differential operators associated with turbulent closures. This will provide a stepping stone for the development of non-local, scale dependent turbulence closures in ocean modeling. It will provide a framework for the creation of a SciServer Database of Canonical Geophysical Flows relevant to dynamical oceanography, in similar spirit to the Johns Hopkins Turbulence Database.
PI: Marc Stein (School of Education, BERC)
Co-I: Julia Burdick-Will (Sociology, KSAS), Gerard Lemson (IDIES)
The overarching goal for this project is to set up the pipeline to develop a “real-time” database of Baltimore transit and crime data on the SciServer platform that can be used to estimate daily routes to school using public transit, estimate daily variation in commuting difficulty (travel time, transfers, delays due unreliable service) and violence exposure on those routes.
PI: Rene Vidal (Biomedical Engineering, WSE)
Co-I: Benjamin Haeffele (MINDS), Matthew Ippolito (Medicine, SOM)
The current proposal will build on computer vision techniques recently developed by Dr. Haeffele in the Vidal Laboratory of the Johns Hopkins Whiting School of Engineering, to detect and classify blood cells in low-resolution lens-free images with a reduced volume of annotated data. This project will extend such computer vision methodology for data mining of malaria microscopy data in patient samples from antimalarial drug trials conducted by the Johns Hopkins Malaria Research Institute at the Johns Hopkins Bloomberg School of Public Health. Linking computer vision-based machine learning algorithms to malaria pharmacology promises to unlock novel insights into the effect of drugs on malaria parasites while establishing a new evaluative tool for the assessment and understanding of malaria and its treatment.
Spring 2019
Brian Camley (Physics & Astronomy, Krieger School of Arts & Sciences)
Andrew Ewald (Cell Biology, School of Medicine)
In developing organisms, groups of cells work together to sense chemical signals, sharing information to make measurements more precisely than any single cell can alone. We will characterize how groups of mammary cells process information by studying organoids made of a mixture of active cells (which always believe they see a signal) and normal cells. Over time, these organoids develop branches, as during normal mammary development. Our plan will be to use the location of the active cells to predict the location of the branches, inferring which cells are most important from experimental data. Understanding how the pattern of activity is translated into branching will allow us to better understand how chemical signals are integrated across a group of cells.
Chris Cannon (English & Classics, Krieger School of Arts & Sciences)
Sayeed Choudhury (Sheridan Libraries)
Mark Patton (Sheridan Libraries)
The history of English meter before 1500 has been difficult to write because we cannot tell from the way poetry was written down how it sounded. Geoffrey Chaucer is the central figure in this story, the inventor of iambic pentameter, the staple of English verse until the 20th century, even though the norms of Middle English grammar suggest that his verse was still sometimes irregular. This project will use a database of all of Chaucer’s words tagged for its grammatical function (and his contemporary John Gower), now tagging each word metrical function—compared throughout with the metrical function of Gower’s words as a control—to ask what happens to Middle English grammar if Chaucer’s verse was always regular.
Sarah Wheelan (Oncology, School of Medicine)
Jai Won Kim (IDIES, Krieger School of Arts & Science)
Jonathon Pevsner (Neurology, Kennedy Krieger Institute)
Luigi Marchionni (Neurology, School of Medicine)
Frederick Tan (Bioinformatics, Carnegie Institution)
We will create a robust platform for teaching students how to execute and interpret nontrivial genomics workflows. We plan to combine our longstanding experience in teaching R and Unix with the flexible and powerful SciServer platform, developed within the IDIES. We will adapt existing content to SciServer and will create new content that leads students through reproducible analysis of truly large-scale datasets, that are realistic examples of what they will encounter in their own work. Explanatory video tutorials will be created as well, enabling independent study.
Scot Miller (Environmental Health & Engineering, Whiting School of Engineering)
Darryn Waugh (Earth & Planetary Sciences, Krieger School of Arts & Sciences)
Methane is the second-most important greenhouse gas and plays a critical role in global climate. Methane mysteriously began to rise in 2007 and has been increasing ever since, implying that methane emissions are also increasing. Scientists do not understand where, how, when, or why emissions changed.
A new satellite promises to fundamentally change methane monitoring. The Sentinel-5 Precursor satellite launched in late 2017 and observes methane with far better global coverage than previous satellites. We plan to create a TROPOMI-based tropospheric methane product and use this product to estimate global methane emissions. This research will elucidate the distribution of global methane, and we can begin to hypothesize which source types are driving emissions, human or natural.
Rajat Mittal (Mechanical Engineering, Whiting School of Engineering)
Andreas Andreou (Pediatrics, School of Medicine)
W. Reid Thompson (Pediatrics, School of Medicine)
Jung Hee Seo (Mechanical Engineering, Whiting School of Engineering)
Wearable sensors are now able to automatically record and analyze our movements, pulse-rates, O2 saturation, sleep and respiration rates. Heart sounds encode vital information about our cardiovascular system, but automated acquisition of these acoustic signals remains a challenge. Recently, our team has developed and tested a novel wearable phonocardiographic (PCG) system, the “StethoVest.” However, effects of body-habitus on PCG measurements and meaningful analysis of the complex signals remains an open issue and is the focus of this project. A multidisciplinary team of mechanical and electrical engineers will combine forces with a cardiologist and employ a suite of tools ranging from patient measurements and computational models, to explore these fundamental questions.
Roman Galperin (Carey Business School)
Marshall Shuler (Neuroscience, School of Medicine)
How do people learn to search for information in unfamiliar domains? What is the role of peers and social context? We aim to improve our understanding of these questions by studying human search behavior in examining innovations. We will apply the insights developed in neuroscience and social sciences to develop a model of social learning of search, using data on hundreds of millions of searches conducted by patent examiners while evaluating inventions. We propose that the examiners’ task of finding specific, relevant knowledge in unfamiliar fields under time constraints represents a general problem of efficient search in knowledge space. We expect that examiners learn to search more efficiently over time and rely on peers for the learning. Our study will contribute to current theories of learning and search for knowledge, produce specific suggestions for improving the patent examination process, and create a dataset for the larger researcher community.
Janet Markle (Molecular Microbiology and Immunology, Bloomberg School of Public Health)
Anthony Guerrerio (Pediatrics, School of Medicine)
This project aims to uncover genetic and immunological drivers of disease pathogenesis in children with very early onset inflammatory bowel disease (VEOIBD). The project combines data-intensive genome-wide sequencing capabilities and cellular immunology expertise with unique patient access. VEOIBD is a rare and devastating disease which may result from single-gene inborn errors of immunity, however most children with this disease currently lack a genetic diagnosis. We propose the in-depth analysis of whole exome sequencing data to identify novel candidate mutations, followed by functional testing of these candidates at the molecular and cellular levels. Through this effort we hope to provide a more complete understanding of VEOIBD pathogenesis on a patient-by-patient level, which will permit tailored therapies in the future.
Spring 2018
Tak Igusa (Center for Systems Science and Engineering, Department of Civil Engineering, Whiting School of Engineering)
Hadi Kharrazi (Center for Population Health Information Technology, Department of Health Policy and Management, Johns School of Public Health)
Autonomous vehicles (AVs) have the potential to transform mobility and reduce the burden of motor vehicle crashes. Before this future can become reality, there is a need for extensive testing of AVs. A key challenge for AV developers is determining the location and timing of AV testing. While AV engineers are mastering factors such as motion control, path planning, localization, perception and mapping, they have not yet considered in suitable depth, the epidemiology of crash risk, particularly within urban settings. In this collaboration between public health and systems engineering, we will develop an epidemiology-based simulation tool, operating within IDIES’ SciServer, that would enable AV R&D to generate high-resolution data of crash risk to inform the development of AV testing programs.
Alexis Battle (Department of Biomedical Engineering, Whiting School of Engineering)
Kasper Hansen (Department of Biostatistics, Bloomberg School of Public Health)
Ali Afshar (Department of Biomedical Engineering, Whiting School of Engineering)
Our project aims to address some of the high-impact research problems in analyzing large-scale vital signs data available through Electronic Health Records. Specifically, our team plans to develop data analytics tools to visualize and interpret time-dependent vital signs data to: 1) Identify patients who experience significant variations in blood pressure for short (few minutes) and/or longer periods of time (several days). These would include, but are not limited to, patients diagnosed with heart failure, a common cause for hospital admission among people over 65 years of age. 2) Determine the relationship between variability in vital signs and clinical outcome. The overall goal of this work is to improve quality of medical care by using data analytics tools that can simplify complex data and better inform clinical decision making.
Seth Blackshaw (Department of Neuroscience, School of Medicine)
Jonathan Ling (Neuroscience, School of Medicine)
RNA sequencing provides an inexpensive, high-resolution window on gene expression patterns. With the accumulation of sequencing data in public archives, researchers now have vast datasets in which to search for clinically-relevant patterns. But the computational resources and skills needed to query the data are not widely available. We will create new software systems enabling large-scale splicing screens against hundreds of thousands of archived samples. The systems will (a) answer queries about splicing associations, e.g. between transcription factors and splicing in disease, and (b) perform bulk screens to find associations between metadata variables (e.g. knock-down or disease states), and splicing patterns. We will use these tools to find associations relevant to neurodegenerative disease and cancer.
Tamas Budavari (Department of Applied Mathematics and Statistics, Whiting School of Engineering)
Our area of investigation begins with attempting to understand the relationship between Twitter’s tweet sentiments by geo-location and the ability of such information to predict stock price movements and risks. An important problem that many investors face originates from not having a good understanding of the true drivers of risks associated with their stock investments. If we better understand the predictive nature of stock prices, then we could provide adequate risk management during turbulent times to insulate such investments from downside deviations. Given the increases in social media activity as evidenced by the proliferation of data generated from Twitter users, coupled with the recent evidence that links do exist between social media data and stock price movement, the natural extension would be to examine the geo-location information available on some tweets. We hypothesis that positive (negative) tweet sentiments around certain key locations will be positively (negatively) correlated with future prices movements. Some of the geo-locations that will be examined in this research include corporate headquarters and high-volume retail stores.
Lingxin Hao (Department of Sociology, Krieger School of Arts and Sciences)
Gerard Lemson (Department of Physics and Astronomy, Krieger School of Arts and Sciences)
Social networks are fundamental in social sciences. The study of social networks, however, has been limited to small networks for three reasons. First, network data scale quadratically with the number of individuals. Second, structural strategic models of network formation and dynamics and agent-based models of social interactions impose complex challenges in estimation. Third, how homophily (the tendency for individuals to connect based on similar characteristics) arises from common unobserved attributes is a new area of research that demands huge computational capacity. In this project we will integrate structural modeling from economics and agent-based models from computational sociology with data-intensive methods developed in the physical sciences to study the dynamics of social networks. We will apply our methods to school friendship networks and migration networks at SciServer and make our simulated data and computational codes available for the research community.
Katherine C. Wu (Department of Medicine, Division of Cardiology, School of Medicine)
Dan M. Popescu (Department of Applied Mathematics and Statistics, , Whiting School of Engineering)
The goal of the research proposed here is to develop and utilize in clinical practice groundbreaking targeted strategies for predicting risk of sudden cardiac death (SCD) from arrhythmias. The proposed research will utilize a novel disease-specific personalized virtual-heart approach combined with machine learning on clinical data to predict the functional electrical behavior of the patient’s heart under a variety of stressor conditions and unmask potential dysfunctions. The robust disease-specific personalized risk assessment approaches proposed here are expected to lead to a radical change in patient stratification for SCD risk and selection for prophylactic implantable defibrillator deployment. This will result in a dramatically improved SCD prevention and in elimination of unnecessary device implantations, engendering precise clinical decision-making regarding personalized treatment.
Mark Dredze (Center for Language and Speech Processing & Malone Center for Engineering in Healthcare, Whiting School of Engineering)
Christopher Chute (School of Medicine & Chief Research Information Officer, Johns Hopkins Health System)
Almost all healthcare interactions are now documented by electronic health records (EHRs). The majority of EHR content is captured as “free-text.” These unstructured data are currently the most complete source of digital information on social determinants of health (SDH). SDH factors are critical for targeting medical and public health interventions. This pilot project will analyze EHR data from cohorts of patients at Atrius Health HMO in Massachusetts and the JH Health System. This project will focus on three research questions; Can SDH information in text be accurately categorized; What is the prevalence of SDH risk factors expressed in these records; and, Can natural language processing (NLP) methods effectively derive SDH information in large EHR free text databases?
Spring 2017
Raman Arora (Department of Computer Science, Whiting School of Engineering)
Currently, scientists have unprecedented access to a wide variety of high quality datasets which are collected from independent studies. However, standardized annotations are essential to perform meta analyses, and this presents a problem as standards are often not used. Accurately combining records from diverse studies requires tedious and error-prone human curation, posing a significant time and cost barrier.
We propose a novel natural language processing (NLP) algorithm, Synthesize, that merges data annotations automatically and is part of an open source web application, Synthesizer, that allows the user to easily interact with merged data visually. The Synthesize algorithm was used to merge varying cancer datasets and to also merge ecological datasets. The algorithm demonstrated high accuracy (on the order of 85-100%) when compared to manually merged data.
Jung Hee Seo (Department of Mechanical Engineering)
Cynthia F. Moss (Psychological and Brain Sciences)
Susanne J. Sterbing-D’Angelo (Psychological and Brain Sciences)
Animals that rely on active sensing provide a powerful system to investigate the neural underpinnings of natural scene representation, as they produce the very signals that inform motor actions. Echolocating bats, for example, transmit sonar signals and process auditory information carried by returning echoes to guide behavioral decisions for spatial orientation. Bats compute the direction of objects from differences in echo intensity, spectrum, and timing at the two ears; while an object’s distance is measured from the time delay between sonar emission and echo return. Together, this acoustic information gives rise to a 3D representation of the world through sound, and measurements of sonar calls and echoes provide explicit data on the signals available to the bat for orienting in space.
In the present seed funding program, we propose to develop a first-of-its-kind computational simulation-enabled method for echo scene analysis of an echolocating bat, which is based on acoustic simulations (we refer to this method as “EchoSIM”). The proposed method integrates tightly with free-flight laboratory assays of bats and takes as input, variables such as the bat’s flight path, hear-ear anatomy, position and orientation as well as the sonar call wave form. The simulation results (3D echo scene and echo signal) together with the experimental measurements will provide a unique and powerful integrated dataset that enable unprecedented analysis of active sensing and adaptive flight behavior of bats in complex environments.
Anand Gnanadesikan ( Department of Earth and Planetary Sciences)
Environmental policy is increasingly based on results from computer simulations, but more integration between models and observations is needed to make sound decisions. For example, the Environmental Protection Agency (EPA)regularly uses models to set the total maximum daily load (TMDL) limits for nutrients entering watersheds, such as the Chesapeake Bay, with the goal of making all waterways in the US fishable and swimmable under the Clean Water Act. Predictions used for policy decisions are typically informed by a series of models, refined by observations and represent input from a variety of scientists.
We propose to optimize the integration of sequence-based approaches into biogeochemical models, with specific application to ChesROMs, a model of the Chesapeake Bay Dead-zone. Run-off from agricultural and urban areas pollutes the Bay surface waters with nitrogen and phosphorous. This pollution drives harmful algal blooms that have devastating consequences on the ecosystems and threaten public health. One major consequence of pollution is the development of oxygen-free (anoxic) or reduced oxygen (hypoxic) dead-zones that deteriorate the habitat for many aquatic animals. An interdisciplinary approach to this problem is essential as the physical environment and microbial processes components are inextricably linked. Physical stratification within the water column, based on salinity and temperature gradients, determine the extent of vertical mixing between the upper and lower water bodies. Microbial processes are sensitive to mixing, adjusting not only growth, but the specific metabolic pathways, based on the amount of mixing. Denitrification and dissimilatory nitrate reduction to ammonia are two processes that can be very sensitive to the physical environment, yet which determines the fate of nitrogen that fuels algal growth. Integrating an understanding of the physical environmental and microbial processes is vital for improved predictions.
Sahan Savas Karatasli (Sociology and Arrighi Center for Global Studies)
Christopher Nealon (Professor and Chair, English Department)
The purpose of the seed proposal is to develop methods to semi-automate the collection of data on protest and other events from newspapers and similar sources with the goal of both reducing the time and increasing the accuracy for coding event information (e.g., location, actors, actions, demands). Most existing social science research in this area automate the data collection process, but do so at the cost of including an unacceptable level of false positives and failing to take advantage of the rich detailed information provided in the newspaper articles themselves. Our current NSF-funded research on Global Social Protest uses search strings to extract relevant articles from the digitized newspaper archives and relies on a custom-built website for data coding and analysis; however, to avoid the above-mentioned pitfalls it relies on human coding of articles (which is time consuming). The seed project seeks to develop natural language processing tools that allow for a middle path between full automation and manual coding. In addition to English language newspapers, we will run pilots on French, Japanese, Korean and Spanish newspapers. The extension of the project to other languages allows us to widen and deepen ongoing international research collaborations.
Spring 2016
Gerard Lemson (Physics & Astronomy)
This seed grant project will pave the way to implementation of an online benchmark ocean circulation solution. In the seed grant we will develop methods and protocols and implement a prototype solution with much smaller data size. The target analytics services are:
- Extraction of sub-spaces of the solution state vector.
- Computation of statistics on the extracted sub-spaces, like time series of heat content in a control volume.
- Computation of oceanographic diagnostics like fluxes of volume, heat, and momentum.
- Computation of conditional statistics, like the temperature on a surface conditioned on strong volume flux.
- Computation of Lagrangian particle trajectories starting from arbitrary initial locations.
Thomas Woolf (Physiology & Computer Science)
Yanif Ahmad (Computer Science)
Dr. Clark’s team is building ‘Metabolic Compass,’ a mobile health stack for investigating circadian rhythms and how our temporal decisions influence near- and long-term health. By tracking when people eat, when they sleep, and when they exercise through Apple’s HealthKit, they will collect a rich, open dataset for studying time restricted feeding and intermittent fasting. Their data will allow users to ask and answer personalized health questions, such as “How much time should I leave between exercising and eating?” or “How early should I eat dinner before going to bed?”. Users will consent through Apple’s ResearchKit, enter data through activity trackers (e.g., FitBit, Jawbone) and third party apps (e.g., MyFitnessPal, Argus), and compare their health against our population through our AWS cloud services. In addition to deploying on iOS, Dr. Clark’s team will explore an Android App to expand our user base during this proposal.
Michael C. Schatz, PhD, (Department of Computer Science)
The non-contiguous nature of eukaryotic coding sequences generates immense protein and RNA diversity from one gene, and poses a challenge for scientists investigating gene function. Short-read sequencing captures tiny snapshots of the immense combinatorial problem; thus, we have likely identified only a small fraction of the functional transcripts in any cell. A novel mechanism is possible: chromatin structure places genes in physical proximity and creates opportunities for RNA-level rearrangements, without corresponding DNA rearrangements. These have been reported anecdotally and would be a mechanism for creating immense transcript diversity. Such transcripts may be detectable only in large and validated datasets, by fast and sensitive algorithms. Longer-read technology, well known to our group, may also be employed.
Carey Priebe, Professor, (Applied Mathematics and Statistics)
This research will aim to deliver new streaming tools to statistical inference on massive graphs as well as address some basic questions in statistics such as hypothesis testing. According to Dr Braverman, the preliminary results indicate that this direction is promising. In particular, it will be able to distinguish between Erdos-Renyi and Kidney-and-Egg random graphs. This novel approach is based on efficient computations of largest eigenvalues of streaming graphs. Dr. Braverman states “We use a combination of measure of concentration tools with streaming algorithms for linear algebra, and we plan to extend these results to more general distributions and submit a white paper in August.”
Spring 2015
Ted Dawson (Neurology (SOM),Neurology (JHH), Neuroscience (SOM))
Valina Dawson (Neurology (SOM), Neuroscience (SOM), Physiology (SOM))
In this project the investigators will develop a data science approach for studying global gene regulation. They will utilize massive amounts of publicly available functional genomic data to build computational models to predict genome-wide cis-regulatory element activities based on gene expression data. The investigators will develop new high-dimensional regression and prediction methods for big data and test the feasibility of predicting cis-regulatory element activities in samples where the available material is insufficient for conventional ChIP-seq and DNase-seq experiments.
Suchi Saria (Dept. of Computer Science)
Advances in model prediction for problems that have a non-trivial cost structure are needed. In healthcare, the financial, nurse time, and wait time costs share a complicated dependency with the clinical measurements needed and medical tests performed. In 2014, the healthcare budget in the United States came to 17% of GDP with a total annual expenditure of $3.1 trillion dollars. It is estimated that between one-fourth and one-third of this amount was unnecessary, with most attributed to avoidable testing and diagnostic costs. Therefore, the design of new cost-sensitive models that faithfully reflect the preferences of a user is paramount. We will develop such models and new optimization algorithms to solve them that give better predictions at lower costs, incorporate a patient’s preferences, and assist in personalized healthcare.
Kathleen Zackowski (Motion Analysis Lab)
The lack of sensitive outcomes capable of detecting progression of Multiple Sclerosis (MS) is a primary limitation to the development of newer therapies. Wearables provide real-time objective measurement of physical activity of MS patients in a real-world context. We put forward a novel statistical framework that simultaneously characterizes multiple features of physical activity profiles over the course of a day as well as their day-to-day dynamics. The proposed framework will allow MS researchers to identify physical activity signatures that will distinguish between individuals with different MS types and will help to understand physical activity differences in disability progression.
Fall 2014
Kathryn Edin (Dept. of Sociology)
Michael Braverman (Dept. of Housing & Community Development, Housing Authority of Baltimore City)
Our Vacant Housing Dynamics in Baltimore City Project aims to improve the quality of city life by integrating data-driven science with redevelopment-policy and administration. Working with City officials, our goal is to better understand the dynamics of vacant housing in Baltimore City, measure the impact of current interventions, and hone decision- and policy-making with statistical analyses of available data. Addressing the vacancy crisis is essential to attracting and retaining people in Baltimore, a key goal formalized in the Grow Baltimore program.
Yanif Ahmad (Dept. of Computer Science)
Raman Arora (Dept. of Computer Science)
The astronomical data space has dramatically increased over the past fifteen years, thanks to detector technology and space-based observations opening up new wavelengths channels. Surprisingly, attempts to characterize and represent the data globally have been rather limited. With this project, we propose to: (i) identify a standard set of operations to look globally at datasets; (ii) explore the potential of various techniques used in statistics and Machine Learning; (iii) define and build efficient tools to conducting global data exploration given one dataset or a combination of them. The goal of this project is to develop a preliminary package allowing a user to perform global data exploration and gain knowledge on the content of the data space.
Rajat Mittal (Dept. of Mechanical Engineering)
Rafael Tamargo (Dept. of Neurosurgery & Otolaryngology)
Justin Caplan (Dept. of Neurosurgery)
Prompt and accurate stratification of rupture risk is the “holy-grail” in treating intracranial aneurysms. Physics-based computational models of aneurysm biomechanics including the simulation of blood flow field and its effect on the vascular structures hold great promise in this context, but large sample sizes are essential for developing insights and reliable statistical correlations/metrics for the rupture risk. In this project, we will develop computational modeling approaches designed from ground-up to process large sample sizes of patient data, that are essential to develop the computer-aided risk stratification method.
Seth Guikema (Dept. of Geography & Engineering )
Dr. Sharon Gourdji (International Center for Tropical Agriculture (CIAT) Cali, Colombia)
One of the greatest challenges in climate science today is the call to provide actionable information for adaptation to climate change. This is a particularly difficult problem because Global Climate Models (GCMs) are poorly suited for predicting climate impacts of interest at local scale. This means that GCM projections must be “downscaled” to the local environment, often through statistical methods. This seed grant is motivated by the recognition that existing statistical downscaling systems suffer from subjective and incomplete selection of predictor fields. To address this limitation we are implementing an automated statistical downscaling system that employs a combination of optimization and statistical learning theory driven predictive modeling. This system will generate predictive models informed by multiple modeling approaches and a diverse and expandable library of gridded predictor fields.
Spring 2014
Raimond Winslow (Dept. Biomedical Engineering)
Yair Amir, (Dept. of Computer Science)
We are designing Sirenic as open-source data streaming infrastructure for the real-time analysis of patient physiological data in intensive care units. Sirenic exploits systems specialization and scaling capabilities enabled by our K3 declarative systems compilation framework to realize orders of magnitude data throughput gains over current generation stream and database systems. Our proposal aims at delivering a proof-of-concept data collection and analysis pipeline to support exploratory research activities in ICU healthcare, with the explicit capability to operate on live data and to empower alarms research and event detection in the real-time setting.
Srinivasan Yegnasubramanian, (Dept. of Oncology)
Alignment to The Cancer Genome Atlas Project Raw Sequencing Reads: With skyrocketing numbers of whole genome sequence and phenotype data available from individuals’ germline and diseased cells, we need a new framework for understanding genomics data. Using the Data-Scope (a data-intensive supercomputer, funded by the NSF), we aim to detect sets of nucleotide-level variations that best classify given phenotypes. Next, we can find covarying or spatially correlated genomic variations across the entire dataset or within phenotypes. Our final goal, and the most powerful application of these data and algorithms, is to use unsupervised methods to delineate genomic variants that discriminate subsets of the data, without regard to phenotypes.
Gregory Eyink (Applied Math and Statistics)
The Elusive Onset of Turbulence And The Laminar-Turbulence Interface: The onset of chaotic fluid motion from an initially laminar, organized state is an intriguing phenomenon referred to as laminar-to-turbulence transition. Early stages involve the amplification of seemingly innocuous small-amplitude perturbations. Once these disturbances reach appreciable amplitudes, they become host to sporadic bursts of turbulence — a chaotic state whose complexity is only tractable by high-fidelity large-scale simulations. By performing direct numerical simulations that resolve the dynamics of laminar-to-turbulence transition in space and time, and storing the full history of the flow evolution, we capture the rare high-amplitude events that give way to turbulence and unravel key characteristics of the laminar-turbulence interface.
Jeffrey Leek, PhD (Dept. of Biostatistics)
Highly Scalable Software for Analyzing Large Collections of RNA Sequencing Data: We are developing a radically scalable software tool, Rail-RNA, for analysis of large RNA sequencing datasets. Rail-RNA will make it easy for researchers to re-analyze published RNA-seq datasets. It will be designed to analyze many datasets at once, applying an identical analysis method to each so that results are comparable. This enables researchers to perform several critical scientific tasks that are currently difficult, including (a) reproducing results from previous large RNA-seq studies, (b) comparing datasets while avoiding bioinformatic variability, (c) studying systematic biases and other effects (e.g lab and batch effects) that can confound conclusions when disparate datasets are combined.
Lori Graham-Brady (Dept. of Civil Engineering)
Professors Daphalapurkar and Graham-Brady of Hopkins Extreme Materials Institute are constructing a massive dynamic-fragmentation database (FragData) for materials undergoing failure in critical applications. They envisage FragData would help expand understanding on the mechanics of failure processes associated with, for example, disruption of asteroids, fragmentation of protection materials under impact, and debris formation of construction materials under catastrophic loading. The idea is to have the database openly accessible, have tools to carry out in situ analysis, and have the database serve as a central platform for other researchers to interpret the massive data from state-of-the-art particle-based and finite-element-based simulation techniques.